Explainable AI (XAI) as a Policy Imperative
- Dell D.C. Carvalho
- Mar 19
- 2 min read
A Real-World Problem
In 2020, a healthcare algorithm used in U.S. hospitals showed racial bias. The program helped decide which patients needed extra care, but it favored white patients over Black patients. Researchers found that the algorithm relied on past healthcare spending as a key factor, assuming that patients who spent more needed more help. However, Black patients often received less treatment due to systemic disparities, leading the AI to underestimate their needs. The algorithm affected about 200 million people, underscoring the dangers of opaque AI systems¹.
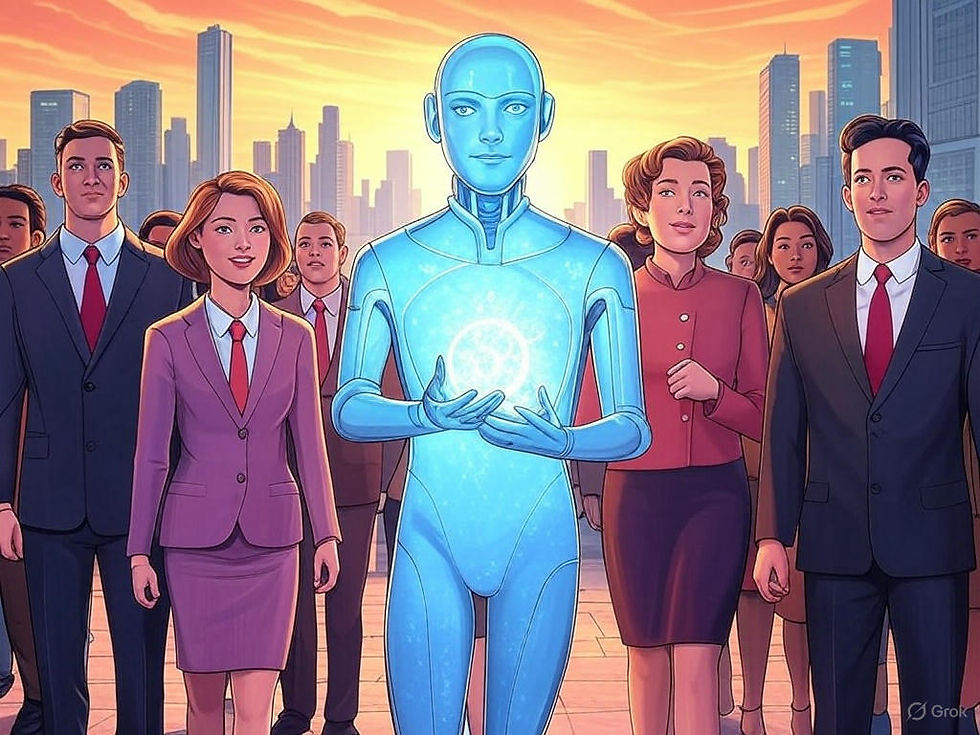
The Need for Explainability
AI affects hiring, lending, healthcare, and criminal justice. When decisions lack transparency, mistakes go unnoticed, and bias spreads. Studies show that 73% of businesses use AI in some way², yet most models function as "black boxes," meaning users cannot see how they reach conclusions. Explainable AI (XAI) allows people to understand AI decisions, ensuring fairness and accountability.
Laws like the European Union’s General Data Protection Regulation (GDPR) require AI decision-making transparency. The U.S. has fewer regulations, but lawmakers are considering similar measures. A 2023 survey found that 81% of Americans want companies to explain AI decisions that affect them³.
Reducing Bias and Errors
Bias in AI can reinforce discrimination. A 2018 study of facial recognition software found error rates of up to 34% for darker-skinned women compared to nearly 1% for lighter-skinned men⁴. Explainable models let researchers identify and fix biases, reducing harm.
Errors in AI decisions can also cause financial losses. A 2022 report found that 60% of businesses using AI suffered from incorrect predictions, leading to lost revenue⁵. XAI helps prevent these costly mistakes by revealing flaws before systems go live.
Conclusion
Explainable AI should be a legal requirement. Without it, biased and flawed AI will continue to harm people. Policymakers must act to ensure AI systems remain fair and transparent.
References
Obermeyer, Z., Powers, B., Vogeli, C., & Mullainathan, S. (2019). "Dissecting racial bias in an algorithm used to manage the health of populations." Science, 366(6464), 447-453.
McKinsey & Company. (2021). "The State of AI in 2021."
Pew Research Center. (2023). "Public Opinion on AI Transparency."
Buolamwini, J., & Gebru, T. (2018). "Gender Shades: Intersectional Accuracy Disparities in Commercial Gender Classification." Proceedings of Machine Learning Research.
Gartner. (2022). "AI Adoption and Business Outcomes."
Comments